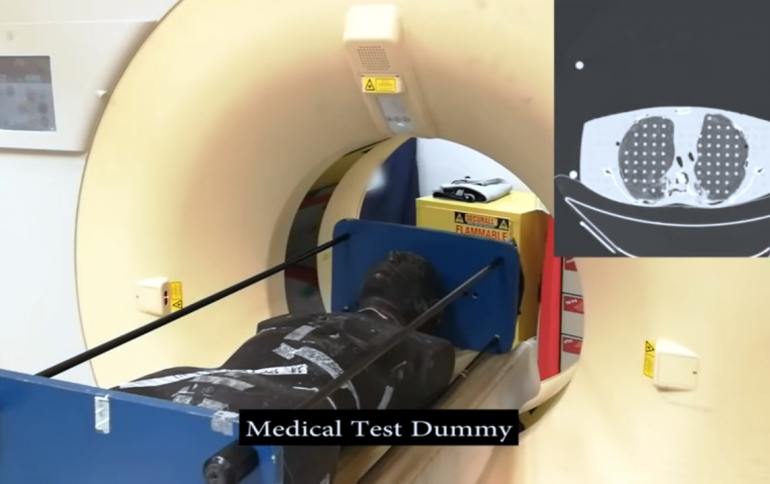
Malware Tricks Radiologists by Removing Cancer Tumors From CT Scans
Researchers in Israel created a malware to prove the security weaknesses in medical imaging equipment and networks.
The researchers, part of the Cyber Security Research Center at Ben-Gurion University in Israel, have developed malware that can automatically alter the imaging before it gets seen by a doctor or radiologist.
They released a demonstration video showing how an attacker can use deep learning to inject or remove lung cancer from CT scans. This attack also affects other 3D medical imagery and medical conditions.
Yisroel Mirsky, Tom Mahler, Ilan Shelef, Yuval Elovici have published a paper that shows how they were able to use "deep-learning to add or remove evidence of medical conditions from volumetric (3D) medical scans."
In their paper, the researchers implement the attack using a 3D conditional GAN and show how the framework (CT-GAN) can be automated. Although the body is complex and 3D medical scans are very large, CT-GAN achieves realistic results which can be executed in milliseconds.
To evaluate the attack, the researchers focused on injecting and removing lung cancer from CT scans. They show how three expert radiologists and a state-of-the-art deep learning AI are highly susceptible to the attack. The researchers also explore the attack surface of a modern radiology network and demonstrate one attack vector: they intercepted and manipulated CT scans in an active hospital network with a covert penetration test.
The researchers used real lung scans and manipulated 70 of them remotely using the malware. The three radiologists misdiagnosed conditions most every time according to the Washington Post which reports cancer was diagnosed 99% of the time where fake cancerous nodules were added and where they were removed by the malware diagnosed patients as being healthy 94% of the time. Following this 'blind' testing, the radiologists were told about the manipulation and given 20 more scans to view, of which 10 had been faked: the added nodules were assumed real 60% of the time, the scans with removed nodules 87% of the time. Worse yet, AI-driven software used to automatically screen such scans was fooled 100% of the time.
This type of attack illustrates the need for data integrity and authenticity controls for healthcare data. Medical systems are designed for use within closed system where the security of data and data transfer is the responsibility of the network.
The security industry has solved this for software updates through code signing, and a similar concept could be applied to healthcare.