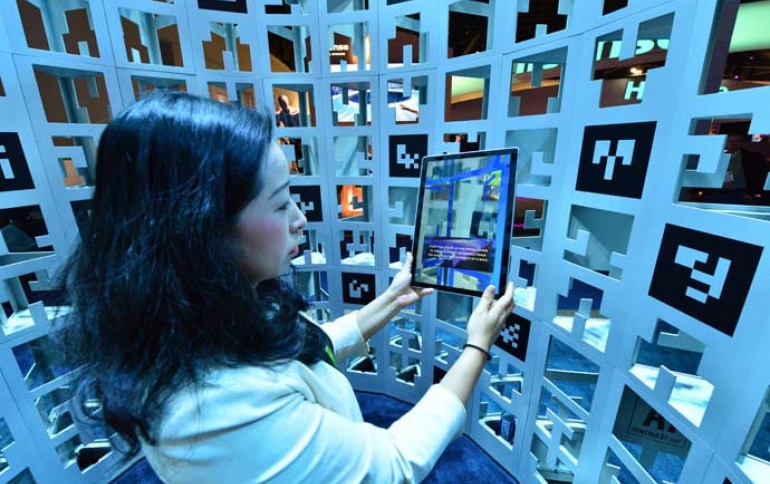
Intel Offers Computer-Powered ‘Echolocation’ Tech and Artificial Intelligence Research at CVPR 2019
Intel is presenting a series of research papers that further the development of computer vision and pattern recognition software and have the potential to transform future technologies across industries – from industrial applications to healthcare to education.
The Intel team presented their research, which leverages artificial intelligence (AI) and ecosystem-sensing technologies to build complete digital pictures of physical spaces, during the Conference on Computer Vision and Pattern Recognition (CVPR), the premier annual computer vision event in Long Beach, California, from June 16-20.
Some of the Intel research presented this week includes:
Seeing Around Corners with Sound
Title: Acoustic Non-Line-of-Sight Imaging by David B. Lindell (Intel Labs), Gordon Wetzstein (Stanford University), Vladlen Koltun (Intel Labs)
In this paper, Intel demonstrates the ability to construct digital images and see around corners using acoustic echoes. Non-line-of-sight (NLOS) imaging technology enables unprecedented capabilities for applications including robotics and machine vision, remote sensing, autonomous vehicle navigation and medical imaging. This acoustic method can reconstruct hidden objects using inexpensive off-the-shelf hardware at longer distances with shorter exposure times compared with the leading alternative NLOS imaging technologies. In this solution, Intel demonstrates how a system of speakers can emit sound waves and leverage microphones that capture the timing of the returning echoes to inform reconstruction algorithms – inspired by seismic imaging – to build a digital picture of a physical object that is hidden from view.
You can watch the demonstration here.
Using ‘Applied Knowledge’ to Train Deep Neural Networks
Title: Deeply-supervised Knowledge Synergy for Advancing the Training of Deep Convolutional Neural Networks by Dawei Sun (Intel Labs), Anbang Yao (Intel Labs), Aojun Zhou (Intel Labs), Hao Zhao (Intel Labs)
AI applications including facial recognition, image classification, object detection and semantic image segmentation leverage technologies inspired by biological neural structures, Deep Convolutional Neural Networks (CNNs), to process information and efficiently find answers. However, leading CNNs are a challenge to train, requiring a large number of hierarchically-stacked parameters for operation, and the more complex they become, the longer they take to train and the more energy they consume. In this paper, Intel researchers present a new training scheme – called Deeply-supervised Knowledge Synergy – that creates “knowledge synergies” and essentially enables the CNN to transfer what it has learned through layers of the network to improve training and performance of CNNs, improving accuracy, noisy data management and data recognition.
Large-Scale Benchmark for 3D Object Understanding
Title: PartNet: A Large-Scale Benchmark for Fine-grained and Hierarchical Part-level 3D Object Understanding by Kaichun Mo (Stanford University), Shilin Zhu (University of California San Diego), Angel X. Chang (Simon Fraser University), Li Yi (Stanford University), Subarna Tripathi (Intel AI Lab), Leonidas J. Guibas (Stanford University), Hao Su (University of California San Diego)
Identifying objects and their parts is critical to how humans understand and interact with the world. For example, using a stove requires not only identifying the stove itself, but also its subcomponents: its burners, control knobs and more. This same capability is essential to many AI vision, graphics and robotics applications, including predicting object functionality, human-object interaction, simulation, shape editing and shape generation. This wide range of applications spurred great demand for large 3D datasets with part annotations. However, existing 3D shape datasets provide part annotations only on a relatively small number of object instances or on coarse, yet non-hierarchical, part annotations, making these datasets unsuitable for applications involving understanding. In other words, if we want AI to be able to make us a cup of tea, large new datasets are needed to better support the training of visual AI applications to parse and understand objects with many small details or with important subcomponents. More information on PartNet here.