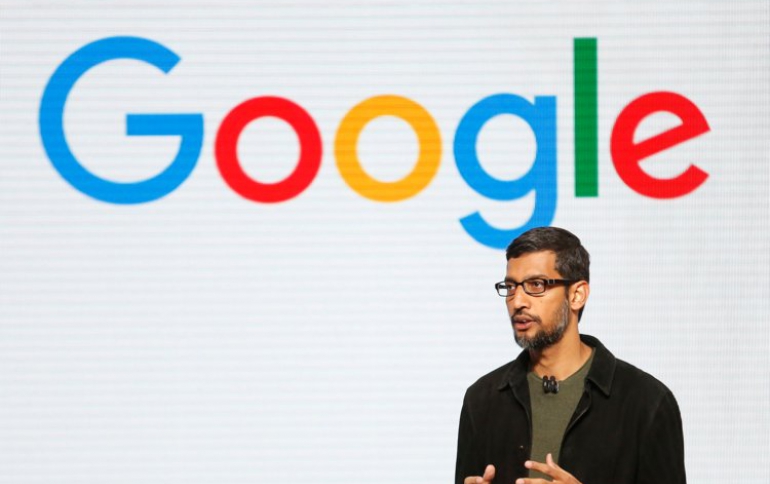
Google's RAISR Algorithm Makes Your Low -quality Pictures Look Sharp and Live
Google has developed a technique that incorporates machine learning in order to produce high-quality versions of low-resolution images. Many of your images are either limited by the resolution of the device used to take the picture, or purposely degraded in order to accommodate the constraints of cell phones, tablets, or the networks to which they are connected. With the ubiquity of high-resolution displays for home and mobile devices, the demand for high-quality versions of low-resolution images, quickly viewable and shareable from a wide variety of devices, has never been greater. With "RAISR: Rapid and Accurate Image Super-Resolution", Googlee introduce sa technique that uses machine learning in order to produce high-quality versions of low-resolution images.
According to Peyman Milanfar, Research Scientist at Google and co-author of a related paper that will appear soon in the IEEE Transactions on Computational Imaging, RAISR produces results that are comparable to or better than the currently available super-resolution methods, and does so roughly 10 to 100 times faster, allowing it to be run on a typical mobile device in real-time. Furthermore, the technique is able to avoid recreating the aliasing artifacts that may exist in the lower resolution image.
Upsampling, the process of producing an image of larger size with significantly more pixels and higher image quality from a low quality image, has been around for quite a while. The common approaches to upsampling are linear methods which fill in new pixel values using simple, and fixed, combinations of the nearby existing pixel values. These methods are fast because they are fixed linear filters (a constant convolution kernel applied uniformly across the image). But what makes these upsampling methods fast, also makes them ineffective in bringing out vivid details in the higher resolution results.
With RAISR, Google instead use machine learning and train on pairs of images, one low quality, one high, to find filters that, when applied to selectively to each pixel of the low-res image, will recreate details that are of comparable quality to the original. RAISR can be trained in two ways. The first is the "direct" method, where filters are learned directly from low and high-resolution image pairs. The other method involves first applying a computationally cheap upsampler to the low resolution image and then learning the filters from the upsampled and high resolution image pairs. While the direct method is computationally faster, the 2nd method allows for non-integer scale factors and better leveraging of hardware-based upsampling. For either method, RAISR filters are trained according to edge features found in small patches of images, - brightness/color gradients, flat/textured regions, etc. - characterized by direction (the angle of an edge), strength (sharp edges have a greater strength) and coherence (a measure of how directional the edge is).
Linear methods simply can not recover the underlying structure, but RAISR can. Below is an example where the aliased spatial frequencies are apparent under the numbers 3 and 5 in the low-resolution original on the left, while the RAISR image on the right recovered the original structure. Another important advantage of the filter learning approach used by RAISR is that it can be specialized to remove noise, or compression artifacts unique to individual compression algorithms (such as JPEG) as part of the training process. By providing it with examples of such artifacts, RAISR can learn to undo other effects besides resolution enhancement, having them "baked" inside the resulting filters.
Super-resolution technology, using one or many frames, has come a long way. Today, the use of machine learning, in tandem with decades of advances in imaging technology, has enabled progress in image processing that yields many potential benefits. For example, in addition to improving digital "pinch to zoom" on your phone, one could capture, save, or transmit images at lower resolution and super-resolve on demand without any visible degradation in quality, all while utilizing less of mobile data and storage plans.