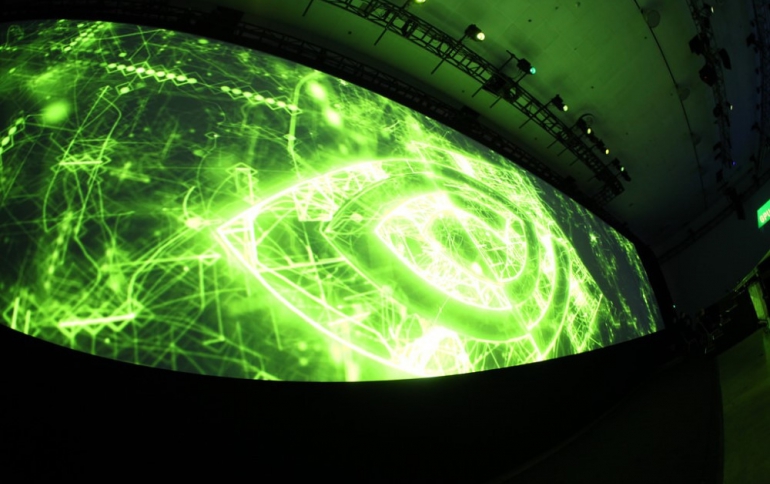
NVIDIA Research Creates 3D Interactive Worlds with AI
NVIDIA today introduced AI research that enables developers for the first time to render entirely synthetic, interactive 3D environments using a model trained on real-world videos.
Speaking at the Conference on Neural Information Processing Systems in Montreal, Nvidia researchers used a neural network to render synthetic 3D environments in real time. Currently, every object in a virtual world needs to be modeled individually using graphics technology, which is expensive and time consuming. In contrast, the NVIDIA research uses "generative" models that automatically learned from real video to render objects such as buildings, trees and vehicles.
The technology offers the potential to quickly create virtual worlds for gaming, automotive, architecture, robotics or virtual reality. The network can, for example, generate interactive scenes based on real-world locations or show consumers dancing like their favorite pop stars.
"NVIDIA has been inventing new ways to generate interactive graphics for 25 years, and this is the first time we can do so with a neural network," said Bryan Catanzaro, vice president of Applied Deep Learning Research at NVIDIA, who led the team developing this work. "Neural networks — specifically generative models — will change how graphics are created. This will enable developers to create new scenes at a fraction of the traditional cost."
The result of the research is a simple driving game that allows participants to navigate an urban scene. All content is rendered interactively using a neural network that transforms sketches of a 3D world produced by a traditional graphics engine into video. This interactive demo was shown at the NeurIPS 2018 conference in Montreal.
The generative neural network learned to model the appearance of the world, including lighting, materials and their dynamics. Since the scene is fully synthetically generated, it can be easily edited to remove, modify or add objects.
Catanzaro said, "Take the example of a face." Generative models would learn not necessarily what an eye or a nose is, but the relationship and positioning of those different features on the face. It includes, for example, how a nose casts a shadow, or how a beard or hair can alter a face’s appearance. Once the generative models have learned a variety of parameters — including how they relate to one another — and how the data is structured, they can generate a synthetic 3D face.
Example sketch-to-face video results. Our method can generate realistic expressions given the edge maps.
"The capability to model and recreate the dynamics of our visual world is essential to building intelligent agents," the researchers wrote in their paper. "Apart from purely scientific interests, learning to synthesize continuous visual experiences has a wide range of applications in computer vision, robotics, and computer graphics," the researchers explained.
Generating a photorealistic video from an input segmentation map video on Cityscapes. Top left: input. Top right:pix2pixHD. Bottom left: COVST. Bottom right: vid2vid (Nvidia's).
This new technology is still in an early-stage research, meaning that you should not expect AI to make Nvidia’s graphics technology obsolete anytime soon.
If all thsese sound interesting to you, you can read the paper released the Nvidia's researchers. The paper's abstract is as folows:
We present a general video-to-video synthesis framework based on conditional Generative Adversarial Networks (GANs). Through carefully-designed generator and discriminator networks as well as a spatio-temporal adversarial objective, we can synthesize high-resolution, photorealistic, and temporally consistent videos. Extensive experiments demonstrate that our results are significantly better than the results by state-of-the-art methods. Its extension to the future video prediction task also compares favorably against the competing approaches.
However, the researchers also say:
Although our approach outperforms previous methods, our model still fails in a couple of situations. For example, our model struggles in synthesizing turning cars due to insufficient information in label maps. We speculate that this could be potentially addressed by adding additional 3D cues, such as depth maps. Furthermore, our model still cannot guarantee that an object has a consistent appearance across the whole video. Occasionally, a car may change its color gradually. This issue might be alleviated if object tracking information is used to enforce that the same object shares the same appearance throughout the entire video. Finally, when we perform semantic manipulations such as turning trees into buildings, visible artifacts occasionally appear as building and trees have different label shapes. This might be resolved if we train our model with coarser semantic labels, as the trained model would be less sensitive to label shapes.