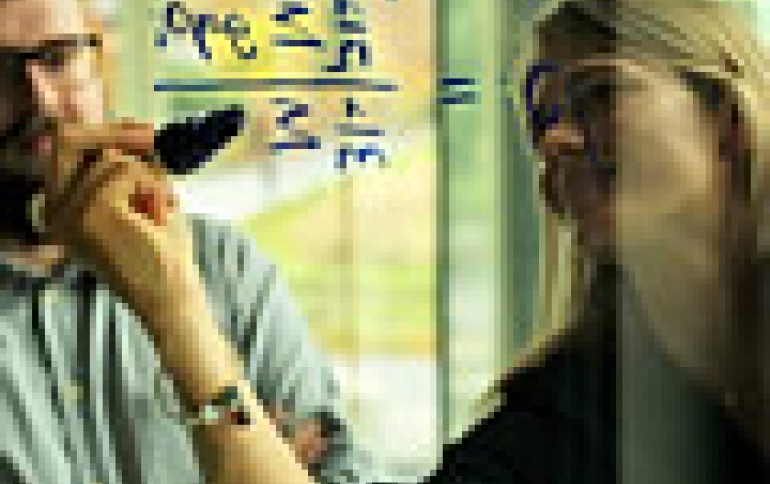
IBM Scientists Demonstrate 10x Faster Machine Learning Using GPUs
Together with EPFL scientists, IBM Research team has developed a scheme for training big data sets quickly - a 10x speedup over existing methods for limited memory training.
The approach, which IBM presented Monday at the Neural Information Processing Systems conference (NIPS 2017) in Long Beach, Calif., uses mathematical duality to cherry-pick the items in a Big Data stream that will make a difference, ignoring the rest. It can process a 30 Gigabyte training dataset in less than one minute using a single graphics processing unit (GPU).
Training a machine learning model on a terabyte-scale dataset is a common, difficult problem. If you're lucky, you may have a server with enough memory to fit all of the data, but the training will still take a very long time. This may be a matter of a few hours, a few days or even weeks.
Specialized hardware devices such as GPUs have been gaining traction in many fields for accelerating compute-intensive workloads, but it's difficult to extend this to very data-intensive workloads.
In order to take advantage of the massive compute power of GPUs, data should be stored inside the GPU memory in order to access and process it. However, GPUs have a limited memory capacity (currently up to 16GB) so this is not practical for very large data.
One straightforward solution to this problem is to sequentially process the data on the GPU in batches. That is, the researchers partition the data into 16GB chunks and load these chunks into the GPU memory sequentially.
Unfortunately, it is expensive to move data to and from the GPU and the time it takes to transfer each batch from the CPU to the GPU can become a significant overhead. In fact, this overhead is so severe that it may completely outweigh the benefit of using a GPU in the first place.
IBM's team set out to create a technique that determines which smaller part of the data is most important to the training algorithm at any given time. For most datasets of interest, the importance of each data-point to the training algorithm is highly non-uniform, and also changes during the training process. By processing the data-points in the right order the researchers can learn their model more quickly.
For example, imagine the algorithm was being trained to distinguish between photos of cats and dogs. Once the algorithm can distinguish that a cat's ears are typically smaller than a dog's, it retains this information and skips reviewing this feature, eventually becoming faster and faster.
This is achieved by deriving novel theoretical insights on how much information individual training samples can contribute to the progress of the learning algorithm. This measure heavily relies on the concept of the duality gap certificates and adapts on-the-fly to the current state of the training algorithm, i.e., the importance of each data point changes as the algorithm progresses.
Taking this theory and putting it into practice the researchers have developed a new, re-useable component for training machine learning models on heterogeneous compute platforms. They call it DuHL for Duality-gap based Heterogeneous Learning. In addition to an application involving GPUs, the scheme can be applied to other limited memory accelerators (e.g. systems that use FPGAs instead of GPUs) and has many applications, including large data sets from social media and online marketing, which can be used to predict which ads to show users. Additional applications include finding patterns in telecom data and for fraud detection.
For their experiments, the researchers used an NVIDIA Quadro M4000 GPU with 8GB of memory training on a 30-Gbyte data set of 40,000 photos using a support vector machine (SVM) algorithm that resolves the images into classes for recognition. The SVM algorithm also creates a geometric interpretation of the model learned (unlike neural networks, which cannot justify their conclusions). IBM's data preprocessing method enabled the algorithm to run in less than a one minute, a tenfold speedup over existing methods using limited-memory training.
The key to the technique is preprocessing each data point to see if it is the mathematical dual of a point already processed. If it is, then the algorithm just skips it, a process that becomes increasingly frequent as the data set is processed.
The next goal for this work is to offer DuHL as a service in the cloud. The researchers are developing the algorithm further for deployment in IBM's BlueMix Cloud, where it will be called Duality-gap-based Heterogeneous Learning.
In a cloud environment, resources such as GPUs are typically billed on an hourly basis. Therefore, if one can train a machine learning model in one hour rather than 10 hours, this translates directly into a very large cost saving.