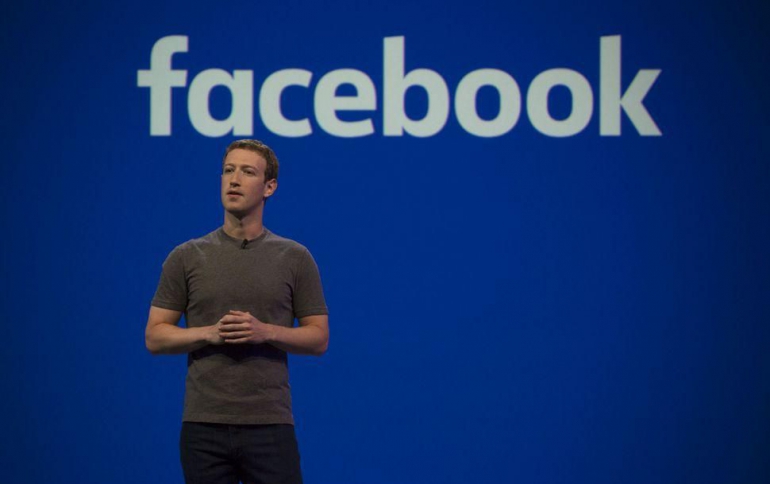
Facebook Confirms Internal Silicon Team
Facebook has confirmed reports the social networking giant is hiring chip engineers and designing at least one ASIC.
Facebook "is absolutely bringing up a silicon team focused on working with silicon providers, and we have a chip we're building, but it's not our primary focus," said Jason Taylor, vice president of infrastructure at Facebook. The chip is "not the equivalent of [Google's] TPU" deep learning accelerator, he added, declining to provide further details on its focus or time frame.
The news came at the @Scale event in San Franscisco, where Facebook announced five chip companies will support Glow, an open-source, deep-learning compiler it backs.
Working with the estimated 50 companies designing AI accelerators is one focus for the new Facebook chip group. "There will be a lot of [accelerator] chips in the market. The big question is whether the workloads they are designed for are the important ones at the time" given it takes two years to field a new chip, Taylor said.
Glow as a generic compiler to let developers target any of the emerging deep-learning accelerators for inference in the cloud or at the edge of the network. It does not target client systems such as smartphones.
"We expect there will be hardware fragmentation [in inference accelerators]. Our work with Glow is to help machine-learning experts design neural nets and not have to do the work required to tune them to each unique chip, Taylor said.
"We know the fragmentation is coming because no one knows what combination of [hardware] resources [such as on-chip memory blocks and multiply-accumulate arrays] will win, so we'll let developers focus on the high-level graphs without hand coding for the specifics of hardware," he added.
Glow takes an AI graph produced by a framework such as TensorFlow or Caffe2 and renders it into byte code for hardware accelerators, Taylor explained. The compiler includes several tools including an instruction scheduler, a linear algebra optimizer, a memory allocator to generate efficient code for a chip's specific memory configuration and a CPU-based reference implementation for testing the accuracy of the hardware.
Cadence, Esperanto Technologies, Intel, Marvell and Qualcomm said they will support Glow on future chips.
Glow is a framework for deploying a neural network in production systems. Its input would be a graph created in a framework such as TensorFlow or Caffe2.
Nvidia's Tensor RT also takes in a graph from a framework and outputs Cuda code for its GPUs.
Facebook, Microsoft and others are backing ONNX, a standard way to express a graph with its weights. In December, the Khronos Group released NNEF, a hardware abstraction layer for deep-learning accelerators.