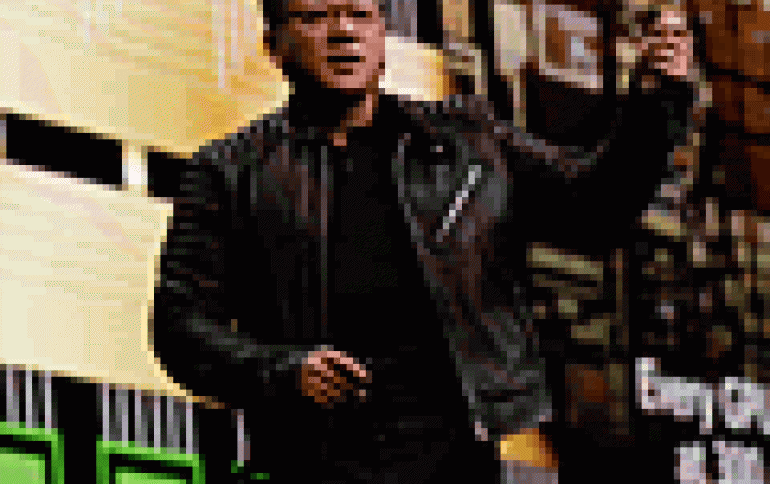
Nvidia Boosts GPU Performance in training Neural Networks, Partners With Arm Partner to Bring Deep Learning to IoT
At its annual GTC event, Nvidia announced enhancements to boost the performance of its GPUs in training neural networks and a partnership with ARM to bring deep learning inferencing to mobile, consumer electronics and Internet of Things devices.
"Clearly the adoption of GPU computing is growing and it's growing at quite a fast rate," NVIDIA CEO Jensen Huang said. "The world needs larger computers because there is so much work to be done in reinventing energy, trying to understand the Earth's core to predict future disasters, or understanding and simulating weather, or understanding how the HIV virus works."
Key advancements to the NVIDIA platform include a 2x memory boost to NVIDIA Tesla V100, the most powerful datacenter GPU, and a new GPU interconnect fabric called NVIDIA NVSwitch, which enables up to 16 Tesla V100 GPUs to simultaneously communicate at a record speed of 2.4 terabytes per second.
The high-end Tesla V100 GPU from Nvidia is now available with 32-GBytes memory, twice the HBM2 stacks of DRAM that it supported when launched last May.
Nvidia became the first company to make the muscular training systems expected to draw 10 kW of power and deliver up to 2 petaflops of performance.
Nvidia launched NVIDIA DGX-2, the first single server capable of delivering two petaflops of computational power. DGX-2 has the deep learning processing power of 300 servers occupying 15 racks of datacenter space, while being 60x smaller and 18x more power efficient.
It is, in effect, a single GPU. "The world wants a gigantic GPU, not a big one, a gigantic one, not a huge one, a gigantic one," Huang said moments before unveiling the DGX-2.
Cray, Hewlett Packard Enterprise, IBM, Lenovo, Supermicro, and Tyan said that they will start shipping systems with the 32-GB chips by June. Oracle plans to use the chip in a cloud service later in the year.
Nvidia said that it trained a FAIRSeq translation model in two days, an eight-fold increase from a test in September using eight GPUs with 16-GBytes memory each. Separately, SAP said that it eked out a 10% gain in image recognition using a ResNet-152 model.
Intel aims to leapfrog Nvidia next year with a production Nervana chip sporting 12 100-Gbit/s links compared to six 25-Gbit/s NVLinks on Nvidia's Volta. The non-coherent memory of the Nervana chip will allow more flexibility in creating large clusters of accelerators, including torus networks, although it will be more difficult to program.
Nvidia currently dominates the training of neural network models in data centers, but it is a newcomer to the broader area of inference jobs at the edge of the network. To bolster its position, Nvidia and ARM agreed to collaborate on making Nvidia's open-source hardware for inferencing available as part of ARM's planned machine-learning products.
So far, ARM has only sketched out its plans for AI chips as part of a broad Project Trillium. An ARM representative would only say that ARM aims to port its emerging neural net software to the Nvidia IP.
TensorRT 4, the latest version of Nvidia's runtime software, boosts support for inferencing jobs and is being integrated into version 1.7 of Google's TensorFlow framework. Nvidia is also integrating the runtime with the Kaldi speech framework, Windows ML, and Matlab, among others.
Separately, the company announced that the RTX software for ray tracing that it announced last week is now available on V100-based Quadro GV100 chips, sporting 32-GBytes memory and two NVLinks.
The software enables faster, more realistic rendering for games, movies, and design models. It runs on Nvidia proprietary APIs as well as Microsoft's DirectX for ray tracing and will support Vulkan in the future.