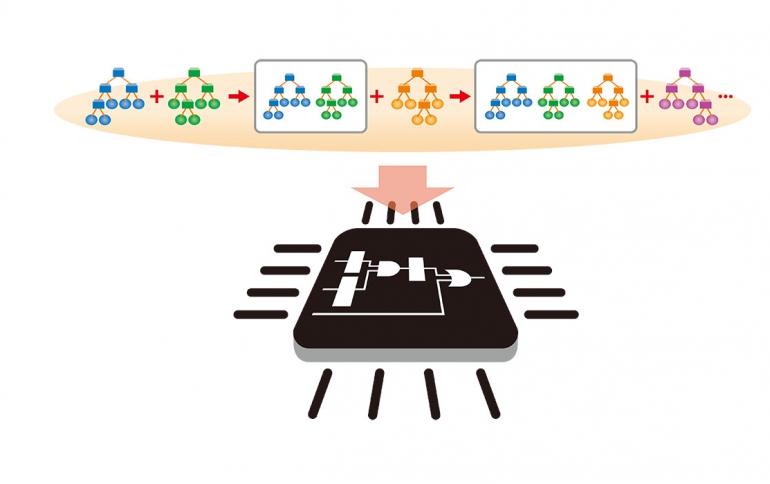
Ricoh Develops Faster and More Power Efficient AI Model Training
Ricoh Company, Ltd. has developed a logic architecture that greatly speeds up Gradient Boosting Decision Tree (GBDT) model training and has significantly lower power consumption compared with traditional methods.
GBDT has been gaining increasing attention in the area of machine training, a form of AI training.
The developed logic architecture was implemented in a Field-Programmable Gate Array (FPGA: an integrated circuit where the designer can change the settings through programming) and the researchers compared training times and power efficiency with three general GBDT software libraries (XGBoost (extreme gradient boosting), LightGBM, CatBoost) using CPU and GPU. They saw that the training speed of the logic architecture on the FPGA was 26-259 times faster compared to the software libraries. In addition, power consumption during the training is low, and the power efficiency of the model training was 90-1,105 times lower compared with general GBDT software libraries using GPU and CPU. This low level of power consumption is especially suited to edge computing. The researchers also confirmed that the prediction accuracy of the trained model is equivalent to that learned from the software libraries.
"GBDT performs highly in the training of large volumes of structured data. We think high-speed training based on the developed logic architecture can contribute to online applications especially in areas such as real-time bidding in online advertisements and recommendations in e-commerce," Rucih said. In finance, the solution can be used in high-frequency trading of financial products by computer and in the security arena, the detection of cyber attacks, as well as in robotics. In addition, the developed logic architecture is suitable for edge devices and computing, which requires low power consumption.
The research group at the Ricoh Institute of Information and Communication Technology announced the results of their study on arXiv.org, a paper contribution website run by Cornell University, USA.