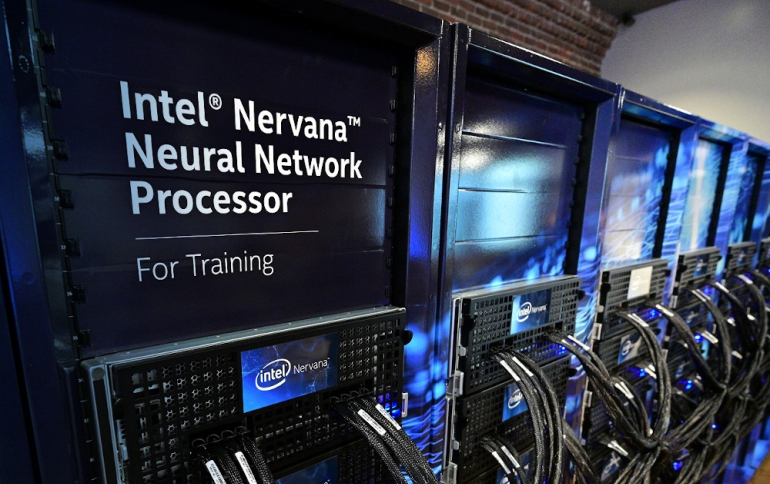
Intel Speeds AI Development With Nervana Neural Network Processors, Intel Movidius Vision Processing Unit
Today at a gathering of industry influencers, Intel welcomed the next wave of artificial intelligence (AI) with updates on new products designed to accelerate AI system development and deployment from cloud to edge.
Complex data, models and techniques are required to advance deep learning reasoning and context, bringing about a need to think differently about architectures.
With most of the world running some part of its AI on Intel Xeon Scalable processors, Intel continues to improve this platform with features like Intel Deep Learning Boost with Vector Neural Network Instruction (VNNI) that bring enhanced AI inference performance across the data center and edge deployments. Intel says it is equipped to look at the full picture of computing, memory, storage, interconnect, packaging and software to maximize efficiency, programmability and ensure the critical ability to scale up distributing deep learning across thousands of nodes to, in turn, scale the knowledge revolution.
Intel provided the first demonstrations of the Intel Nervana Neural Network Processor for Training (NNP-T) and the Intel Nervana Neural Network Processor for Inference (NNP-I). The company also demonstrated for the first time enhanced integrated AI acceleration with bfloat16 on the next-generation Intel Xeon Scalable processor with Intel Deep Learning Boost (Intel DL Boost), codenamed Cooper Lake. Finally, Intel announced the future Intel Movidius Vision Processing Unit (VPU), codenamed Keem Bay, for edge media, computer vision and inference applications.
The Intel Nervana Neural Network Processors (NNP) for training (NNP-T1000) and inference (NNP-I1000) are Intel’s first purpose-built ASICs for complex deep learning with scale and efficiency for cloud and data center customers.
These products further strengthen Intel’s portfolio of AI solutions, which is expected to generate more than $3.5 billion in revenue in 2019.
Now in production, the new Intel Nervana NNPs are part of a systems-level AI approach offering a full software stack developed with open components and deep learning framework integration for maximum use.
Intel says that the Nervana NNP-T purpose-built deep learning architecture carefully balances compute, memory & interconnect near-linear scaling – up to 95% scaling with Resnet-50 & BERT as measured on 32 cards – to train even the most complex models at high efficiency. As a highly energy-efficient compute platform for training real-world deep learning applications, Intel Nervana NNP-T ensures no loss in communications bandwidth when moving from an 8-card in-chassis system to a 32-card cross-chassis system, with the same data rate on 8 or 32 cards for large (128 MB) message sizes, scaling well beyond 32 cards.
For deep learning training models such as BERT-large, Transformer-LT with large weight sizes (> 500MB), Intel Nervana NNP-T systems with simplified glueless, peer-to-peer scaling fabric is projected to have no loss in bandwidth, scaling from a few cards to thousands of cards .
The Intel Nervana NNP-I is power- and budget-efficient, designed for running intense, multimodal inference at real-world scale using flexible form factors.
It is a highly-programmable accelerator platform specifically designed for ultra-efficient multi-modal inferencing. Intel Nervana NNP-I will be supported by the OpenVINO Toolkit, incorporates a full software stack including popular deep learning frameworks, and offers a comprehensive set of reliability, availability, and serviceability (RAS) features to facilitate deployment into existing data centers.
Both products were developed for the AI processing needs of Intel's AI customers like Baidu and Facebook.
Additionally, Intel’s next-generation Intel Movidius VPU (code-named Keem Bay), scheduled to be available in the first half of 2020, incorporates efficient architectural advances that are expected to deliver leading performance — more than 10 times the inference performance as the previous generation — with up to six times the power efficiency of competitor processors, according to Intel.
According to Intel, early performance testing indicates that Keem Bay will offer more than 4x the raw inference throughput of NVIDIA’s similar range TX2 SOC, at 1/3 less power, and nearly equivalent raw throughput to NVIDIA’s next higher class SOC, NVIDIA Xavier, at 1/5th the power. This is in part because of Keem Bay’s mere 72mm2 size vs NVIDIA Xavier’s 350mm, highlighting the efficiency that this new product’s architecture delivers. Keem Bay will also be supported by Intel’s OpenVINO Toolkit at launch and will be incorporated into Intel’s newly announced Dev Cloud for the Edge, which launches today and allows you to test your algorithms on any Intel hardware solution to try before you buy.
Intel introduced Intel Advanced Vector Extensions 512 (Intel AVX-512) instructions in 2017 with the first generation Intel Xeon Scalable processor. With the 2nd Generation Intel Xeon Scalable processor, the company introduced Intel DL Boost’s Vector Neural Network Instructions (VNNI), which combine three instruction sets into one while enabling INT8 deep learning inference.
At the AI Summit, Intel demonstrated how is it improving on this foundation in its next-generation Intel Xeon Scalable processors with bfloat16, a new numerics format supported by Intel DL Boost. bfloat16 is advantageous in that it has similar accuracy to the more common FP32 format, but with a reduced memory footprint that can lead to significantly higher throughput for deep learning training and inference on a range of workloads.
Compute at the network edge requires efficiency and scalability across a broad range of applications and with AI inference requirements come even tighter energy constraints – as low as just a few watts. To best support future edge AI use cases, we’re excited to announce the future Intel Movidius VPU (code-named Keem Bay), releasing in the first half of 2020. Keem Bay builds on the success of our popular Intel Movidius Myriad™ X VPU while adding groundbreaking and unique architectural features that provide a leap ahead in both efficiency and raw throughput.
Early performance testing indicates that Keem Bay will offer more than 4x the raw inference throughput of NVIDIA’s similar range TX2 SOC, at 1/3 less power, and nearly equivalent raw throughput to NVIDIA’s next higher class SOC, NVIDIA Xavier, at 1/5th the power [5]. This is in part because of Keem Bay’s mere 72mm2 size vs NVIDIA Xavier’s 350mm [6], highlighting the efficiency that this new product’s architecture delivers. Keem Bay will also be supported by Intel’s OpenVINO Toolkit at launch and will be incorporated into Intel’s newly announced Dev Cloud for the Edge, which launches today and allows you to test your algorithms on any Intel hardware solution to try before you buy.
Intel’s solution portfolio integrates the compute architectures that analysts predict will be required to realize the full promise of AI: CPUs, FPGAs, ASICs like those Itnel announced today, all enabled by an open software ecosystem.