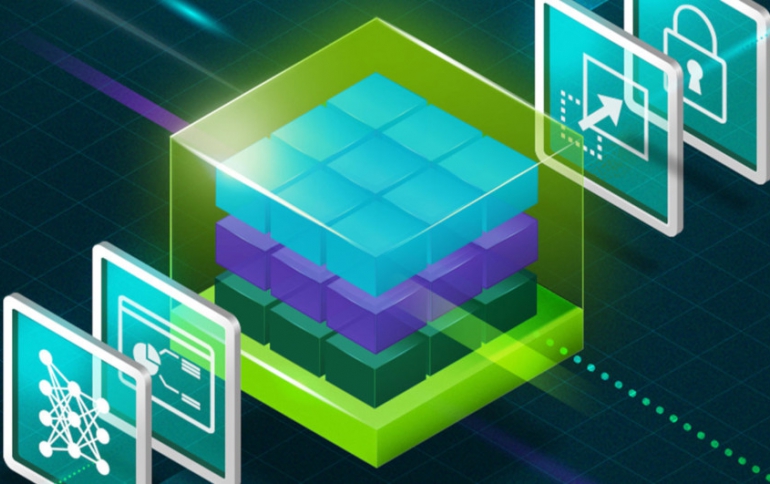
NVIDIA and VMware to Accelerate Machine Learning on VMware Cloud on AWS
Nvidia on Monday said it was teaming up with VMWare and Amazon.com’s cloud division to court large businesses looking to host artificial intelligence programs in Amazon’s cloud.
NVIDIA and VMware plan to deliver accelerated GPU services for VMware Cloud on AWS to power enterprise applications, including AI, machine learning and data analytics workflows. These services will enable enterprises to migrate VMware vSphere-based applications and containers to the cloud, unchanged, where they can be modernized to take advantage of high-performance computing, machine learning, data analytics and video processing applications.
Enterprises are adopting AI and implementing new AI strategies that require powerful computers to create predictive models from petabytes of corporate data. Across industries, enterprises are implementing machine learning applications such as image and voice recognition, advanced financial modeling and natural language processing using neural networks that rely on NVIDIA GPUs for faster training and real-time inference. Additionally, VMware recently acquired Bitfusion, which enables VMware to make GPU capabilities efficiently available for AI and machine learning workloads in the enterprise.
Through this partnership, VMware Cloud on AWS customers will gain access to a new, scalable and secure cloud service consisting of Amazon EC2 bare metal instances to be accelerated by NVIDIA T4 GPUs and new NVIDIA Virtual Compute Server (vComputeServer) software.
Once available businesses will be able to leverage an enterprise-grade hybrid cloud platform to accelerate application modernization. They will be able to unify deployment, migration and operations across a consistent VMware infrastructure from data center to the AWS cloud in support of most compute-intensive workloads, including AI, machine learning and data analytics.
- Customers will be able to move workloads powered by NVIDIA vComputeServer software and GPUs with a single click of a button and no downtime using VMware HCX.
- Elastic AWS infrastructure: With the ability to automatically scale VMware Cloud on AWS clusters accelerated by NVIDIA T4, administrators will be able to grow or shrink available training environments depending on the needs of their data scientists.
- Accelerated computing for modern applications: NVIDIA T4 GPUs feature Tensor Cores for acceleration of deep learning inference workflows. When these are combined with vComputeServer software for GPU virtualization businesses have the flexibility to run GPU-accelerated workloads like AI, machine learning and data analytics in virtualization environments for improved security, utilization and manageability.
- Consistent Hybrid Cloud Infrastructure and Operations: With VMware Cloud on AWS, organizations can establish consistent infrastructure and consistent operations across the hybrid cloud, leveraging VMware industry-standard vSphere, vSAN and NSX as a foundation for modernizing business-critical applications. IT operators will be able to manage GPU-accelerated workloads within vCenter, right alongside GPU-accelerated workloads running on vSphere on-premises.
- The NVIDIA T4 data center GPU supercharges mainstream servers and accelerates data science techniques using NVIDIA RAPIDS, a collection of
- NVIDIA GPU acceleration libraries for data science including deep learning, machine learning and data analytics.
The companies did not say when the software would be released.
NVIDIA vComputeServer
NVIDIA’s virtual GPU (vGPU) technology, now supports server virtualization for AI, deep learning and data science.
Previously limited to CPU-only, AI workloads can now be deployed on virtualized environments like VMware vSphere with new vComputeServer software and NVIDIA NGC. Through Nvidia's partnership with VMware, this architecture will help organizations to migrate AI workloads on GPUs between customer data centers and VMware Cloud on AWS.
vComputeServer gives data center administrators the option to run AI workloads on GPU servers in virtualized environments for improved security, utilization and manageability. IT administrators can use hypervisor virtualization tools like VMware vSphere, including vCenter and vMotion, to manage all their data center applications, including AI applications running on NVIDIA GPUs.
By expanding the vGPU portfolio with NVIDIA vComputeServer, NVIDIA is adding support for data analytics, machine learning, AI, deep learning, HPC and other server workloads. The vGPU portfolio also includes virtual desktop offerings — NVIDIA GRID Virtual PC and GRID Virtual Apps for knowledge workers and Quadro Virtual Data Center Workstation for professional graphics.
NVIDIA vComputerServer provides features like GPU sharing, so multiple virtual machines can be powered by a single GPU, and GPU aggregation, so one or multiple GPUs can power a virtual machine.
Features of vComputeServer include:
- GPU Performance: Up to 50x faster deep learning training than CPU-only, similar performance to running GPU on bare metal.
- Advanced compute: Error correcting code and dynamic page retirement prevent against data corruption for high-accuracy workloads.
- Live migration: GPU-enabled virtual machines can be migrated with minimal disruption or downtime.
- Increased security: Enterprises can extend security benefits of server virtualization to GPU clusters.
- Multi-tenant isolation: Workloads can be isolated to securely support multiple users on a single infrastructure.
- Management and monitoring: Admins can use the same hypervisor virtualization tools to manage GPU servers, with visibility at the host, virtual machine and app level.
- Broad Range of Supported GPUs: vComputeServer is supported on NVIDIA T4 or V100 GPUs, as well as Quadro RTX 8000 and 6000 GPUs, and prior generations of Pascal-architecture P40, P100 and P60 GPUs.
NVIDIA NGC, the company's hub for GPU-optimized software for deep learning, machine learning and HPC, offers over 150 containers, pre-trained models, training scripts and workflows to accelerate AI from concept to production, including RAPIDS, a CUDA-accelerated data science software.
RAPIDS offers a range of open-source libraries to accelerate the entire data science pipeline, including data loading, ETL, model training and inference.
All NGC software can be deployed on virtualized environments like VMware vSphere with vComputeServer.
IT administrators can use hypervisor virtualization tools like VMware vSphere to manage all their NGC containers in VMs running on NVIDIA GPUs.
In addition, NVIDIA helps IT roll out GPU servers faster in production with validated NGC-Ready servers. And enterprise-grade support provides users and administrators with direct access to NVIDIA’s experts for NGC software, minimizing risk and improving productivity.
Dell, Cisco and VMware have shown support for NVIDIA vComputeServer, which is available starting in August.