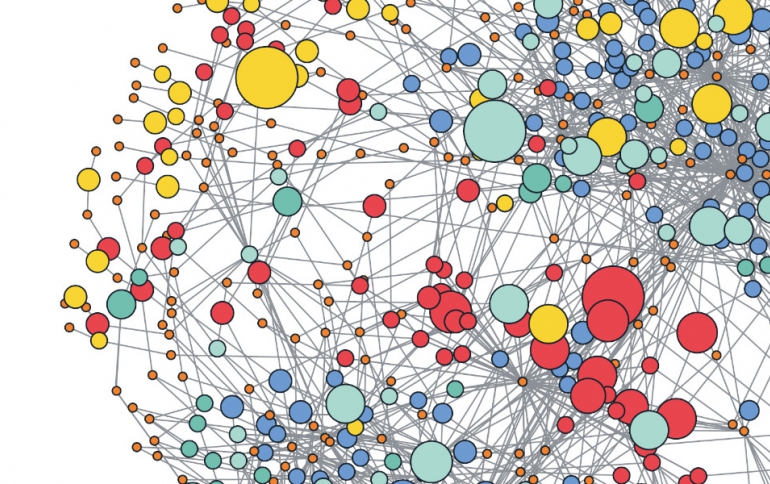
Artificial Intelligence Could Fight Future Diseases
Finding a cure for new diseases requires time and significant investments, but scientists are already looking in artificial intelligence in order to reach to their goals faster.
While it’s probably too late for technology to play a major role in the current Coronavirus epidemic, there’s hope for the next outbreaks. AI is good at combing through mounds of data to find connections that make it easier to determine what kinds of treatments could work or which experiments to pursue next.
Researchers managed to produce the gene sequencing of the Coronavirus within weeks of the first reported cases, showing there’s far more immediate data available now when outbreaks happen.
Cambridge-based Healx is among the companies working to help train artificial intelligence for drug discovery. The company's AI platform, Healnet, uses natural language processing (NLP) to extract disease knowledge from published sources and to complement biomedical databases and proprietary, curated data. It constantly augments the depth and breadth of previously held information and fills in gaps in the knowledge of rare diseases, enbling s greater opportunity for novel treatment discovery.
The often-complex biology of rare diseases means that it is no longer effective to start with a single target in mind. Healx 's approach is hypothesis-free: Healnet leverages the breadth of its dataset, along with disease- and/or drug-specific data, to deliver reasoned insights we would never have thought to consider.
Healnet’s data is integrated in the form of the largest, rare disease-focused Knowledge Graph. It shows prioritised hidden and novel connections between drugs and diseases when explored by expert pharmacologists and biologists. Healnet can present a set of pre-formed, data-driven hypotheses, which speeds up the interpretation process.
Speaking to Bloomberg, Neil Thompson, Healx’s chief science officer, said the company's technique could be deployed against an outbreak like the coronavirus as long as it had enough data on the new disease. Healx isn’t working on tackling the coronavirus or tweaking its technology for outbreaks, but it wouldn’t be a stretch.
“We’re quite close,” Thompson said in an interview. “We wouldn’t need to change much about the AI algorithms we use. We look at matching drug properties to disease features.”
In UK-based Exscientia's approach, dubbed the Centaur Chemist, drug designers help teach the algorithms strategies for searching for compounds. Healx puts the AI’s predictions to researchers who analyze the results and decide what to pursue.
The origin of the term Centaur lies in the first man vs. machine events in chess invented by grandmaster Garry Kasparov after proposing the cooperation of human chess player and machine. The result was a combination of human and machine able to beat both human experts and machines alone. Ultimately, the deciding factor is the process used to combine human and machine.
However the rules of drug discovery are very complex - not all are known and some are not readily describable as a finite set of moves. Therefore it is very challenging to devise a pure machine process for drug discovery.
At Exscientia the Centaur Chemist platform controls the entire process, not single tasks. Through this, learnings about drug discovery moves & rules improve with each project and cycle. This encoded knowledge helps the system become more efficient, and through this approach the technology platform workflows define and execute these design tasks allowing the Centaur designers to define workflows and concentrate on strategy.
Andrew Hopkins, chief executive officer of Exscientia Ltd. is among those working to help train artificial intelligence for drug discovery. He figures new treatments could go from conception to clinical testing in as little as 18 to 24 months within the next decade, thanks to AI.
Researchers at the Massachusetts Institute of Technology said on Thursday that they’d used the method to identify a powerful new antibiotic compound that could kill an array of troublesome bacteria, even some that are currently resistant to other treatments.
Using a machine-learning algorithm, MIT researchers have identified a powerful new antibiotic compound. In laboratory tests, the drug killed many of the world’s most problematic disease-causing bacteria, including some strains that are resistant to all known antibiotics. It also cleared infections in two different mouse models.
The computer model, which can screen more than a hundred million chemical compounds in a matter of days, is designed to pick out potential antibiotics that kill bacteria using different mechanisms than those of existing drugs.
“We wanted to develop a platform that would allow us to harness the power of artificial intelligence to usher in a new age of antibiotic drug discovery,” says James Collins, the Termeer Professor of Medical Engineering and Science in MIT’s Institute for Medical Engineering and Science (IMES) and Department of Biological Engineering. “Our approach revealed this amazing molecule which is arguably one of the more powerful antibiotics that has been discovered.”
In their new study, the researchers also identified several other promising antibiotic candidates, which they plan to test further. They believe the model could also be used to design new drugs, based on what it has learned about chemical structures that enable drugs to kill bacteria.
“The machine learning model can explore, in silico, large chemical spaces that can be prohibitively expensive for traditional experimental approaches,” says Regina Barzilay, the Delta Electronics Professor of Electrical Engineering and Computer Science in MIT’s Computer Science and Artificial Intelligence Laboratory (CSAIL).
The researchers designed their model to look for chemical features that make molecules effective at killing E. coli. To do so, they trained the model on about 2,500 molecules, including about 1,700 FDA-approved drugs and a set of 800 natural products with diverse structures and a wide range of bioactivities.
Once the model was trained, the researchers tested it on the Broad Institute’s Drug Repurposing Hub, a library of about 6,000 compounds. The model picked out one molecule that was predicted to have strong antibacterial activity and had a chemical structure different from any existing antibiotics. Using a different machine-learning model, the researchers also showed that this molecule would likely have low toxicity to human cells.
This molecule, which the researchers decided to call halicin, has been previously investigated as possible diabetes drug. The researchers tested it against dozens of bacterial strains isolated from patients and grown in lab dishes, and found that it was able to kill many that are resistant to treatment, including Clostridium difficile, Acinetobacter baumannii, and Mycobacterium tuberculosis. The drug worked against every species that they tested, with the exception of Pseudomonas aeruginosa, a difficult-to-treat lung pathogen.
To test halicin’s effectiveness in living animals, the researchers used it to treat mice infected with A. baumannii, a bacterium that has infected many U.S. soldiers stationed in Iraq and Afghanistan. The strain of A. baumannii that they used is resistant to all known antibiotics, but application of a halicin-containing ointment completely cleared the infections within 24 hours.
Preliminary studies suggest that halicin kills bacteria by disrupting their ability to maintain an electrochemical gradient across their cell membranes. This gradient is necessary, among other functions, to produce ATP (molecules that cells use to store energy), so if the gradient breaks down, the cells die. This type of killing mechanism could be difficult for bacteria to develop resistance to, the researchers say.
“When you’re dealing with a molecule that likely associates with membrane components, a cell can’t necessarily acquire a single mutation or a couple of mutations to change the chemistry of the outer membrane. Mutations like that tend to be far more complex to acquire evolutionarily,” Stokes says.
In this study, the researchers found that E. coli did not develop any resistance to halicin during a 30-day treatment period. In contrast, the bacteria started to develop resistance to the antibiotic ciprofloxacin within one to three days, and after 30 days, the bacteria were about 200 times more resistant to ciprofloxacin than they were at the beginning of the experiment.
The researchers plan to pursue further studies of halicin, working with a pharmaceutical company or nonprofit organization, in hopes of developing it for use in humans.
One problem that remains in place is clinical testing. Even drugs already safe for use to cure one ailment should be tested again before they’re prescribed for another. The process of showing they are safe and effective on a large number of people can take years before going to regulators for review.
To be effective, AI-based drug developers would have to plan ahead of time, picking out a virus genome likely to cause problems in the future and targeting it when there are few incentives to do so.