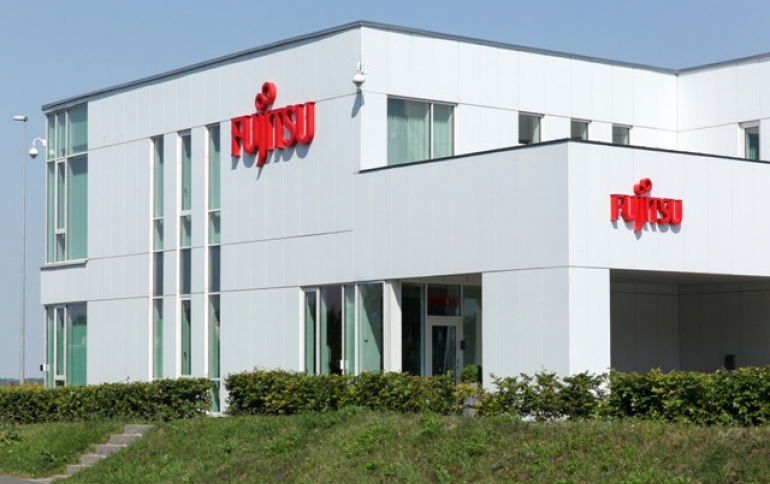
Fujitsu Technologies Accelerate AI Processing by 10 Fold, Ensure High-Accuracy AI Operations
Fujitsu Laboratories, Ltd. has developed new "Content-Aware Computing" technology that can control accuracy while increasing computing speeds. The lad also developed a "High Durability Learning" technology that estimates the accuracy of AI and automatically restores AI models.
Content-Aware Computing
The technology was developed in response to the increasing demand for computing power accompanying the evolution and popularization of AI technologies. Applying this new technology to deep learning tasks promises to accelerate computing speeds by up to ten times, making it easier to utilize AI for an increasing variety of future applications.
In recent years, the spread of AI technologies in areas like image recognition and speech translation has contributed to increasing demands for processing power, straining existing technologies to their limits. New types of GPUs and specialized processors optimized for AI applications of this nature have been developed in response to this trend. AI tasks are calculated in variety of environments depending on the specific application, ranging from cloud environment to edge computing contexts. Technologies that offer both stability and fast processing will prove increasingly necessary to deliver the computing power required to deal with demanding AI tasks.
Graphics processing units (GPUs) and dedicated processors have improved computing performance, but they have not caught up with the computational demands of AI. As a means of further streamlining performance for AI tasks, there is an interest in technologies that lessen computational burden and increase speed. In calculating neural network algorithms in deep learning, for instance, one method for achieving higher performance speeds is to reduce the operation precision from 32 bits to 8 bits and carrying out parallel operations 4 times. Unfortunately, the operation result also deteriorates if the operation precision is uniformly lowered. For this reason, a trained expert must painstakingly determine which area's calculation accuracy should be reduced by trial and error. This method proves time-consuming to adjust, requiring readjustment whenever the input data or execution environment changed.
To overcome these challenges, Fujitsu has developed "Content-Aware Computing" technology that automatically controls and speeds up calculation accuracy. This allows for faster AI processing in a variety of execution environments, including GPUs, CPUs, clouds, and edges.
Neural networks generally have a numerical range in which each layer converges to a similar numerical value as the learning progresses. Based on the distribution of the numerical range of each layer in the neural network during calculation, the degree of application of the calculation accuracy is determined according to the learning situation, such as wide bit width when the distribution is wide, and narrow bit width when the learning is advanced and converged. This allows deep learning to be up to three times faster than before while reducing the degradation of calculation results.
In a cloud environment or other environment where many applications share the CPU, there may be a significant delay in response on some nodes due to communication conflicts, interrupted handling, etc. On the other hand, in each operation of parallel processing, the amount of reduction in processing time when the processing is terminated and the degree of influence on the operation result are estimated, and the termination time of each operation is controlled so that the processing time can be reduced to the maximum extent without deteriorating the operation result. This enables faster parallel processing, leading to confirmed performance of up to 3.7 times faster in deep learning calculations.
Fujitsu says that ultimately, the newly developed technology "Content-Aware Computing" can accelerate processing for AI tasks by up to 10 times. By incorporating this technology into AI frameworks and libraries, it becomes possible to speed up AI processing in cloud environments and data centers using GPUs and CPUs with built-in low-bit computing functions.
Fujitsu aims to incorporate this technology into an AI framework that is widely used and will serve as a foundation for the execution of AI services using deep learning.
High-Accuracy AI Operations
Fujitsu Laboratories, Ltd., has also developed a "High Durability Learning" technology that estimates the accuracy of AI and automatically restores AI models.
In a business context, AI models often struggle to maintain accuracy over the course of operations due to frequent changes in input data caused by shifts in social conditions and market trends. This makes it essential to periodically check the accuracy of AI models being used for business. If deterioration of the accuracy is confirmed, the AI model must be retrained using the latest data to restore the accuracy of its predictions.
Fujitsu says that the newly developed technology makes it possible to automatically estimate the accuracy of the AI model during operations while preventing deterioration of its accuracy.
Using financial data from 3,800 companies, Fujitsu has verified our assessment of credit risk in the financial sector. This technology has made it possible to estimate the accuracy of AI model within a 3% error margin. We also confirmed that this technology can maintain the accuracy of AI at 89%, which was at 69% with the conventional technology.
The new technology can maintain the accuracy of AI model over a long period, delivering reliable and stable operations for users. Fujitsu anticipates that its High Durability Learning technology will ultimately improve the efficiency of AI models not only in the financial sector but in various other businesses, as well, including for product classification in the retail sector and character recognition in the distribution and logistics sector.
Fujitsu aims to incorporate this technology into its goal-oriented ”Design the Trusted Future by Data × AI” processes and framework, as well as for machine learning technologies in its Zinrai portfolio, and aiming to deliver practical use cases by the end of FY 2020.