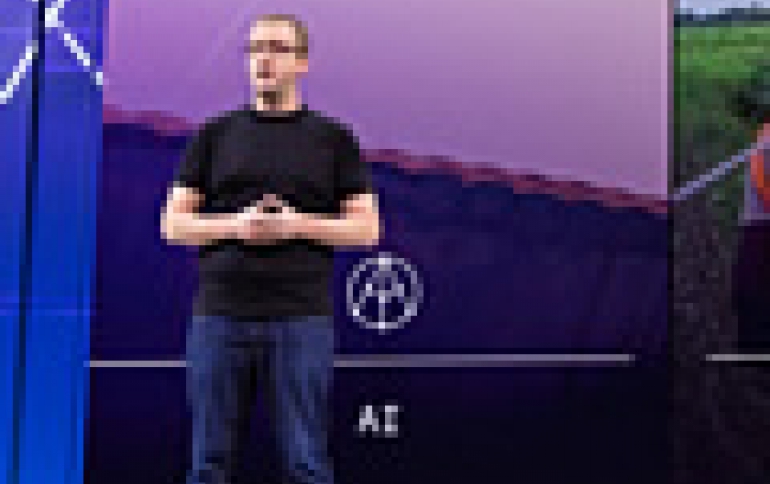
Facebook Advances Image Recognition With Deep Learning on Hashtags
The second day of F8 event focused on connectivity, AI, and AR/VR, and Facebook's researchers announced that they trained an image recognition system on a data set of 3.5 billion publicly available photos, using the hashtags on those photos in place of human annotations.
This new technique will allow researchers to scale their work much more quickly, and they've already used it to score a record-high 85.4% accuracy on the widely used ImageNet benchmark. Facebook said it is already leveraging this work in production to improve the social network's ability to identify content that violates its policies.
This research offers important insight into how to shift from supervised to weakly supervised training, where Facebook uses existing labels - in this case, hashtags - rather than ones that are chosen and applied specifically for AI training.
In the immediate future, Facebook envisions other ways to use hashtags as labels for computer vision. Those could include using AI to better understand video footage or to change how an image is ranked in Facebook feeds. Hashtags could also help systems recognize when an image falls under not only a general category but also a more specific subcategory. For example, an audio caption for a photo that mentions a bird in a tree is useful, but a caption that can pinpoint the exact species, such as a cardinal perched in a sugar maple tree, provides visually impaired users with a significantly better description.
This image recognition work is powered by Facebook's AI research and production tools: PyTorch, Caffe2, and ONNX. Facebook announced the next version of its open source AI framework, PyTorch 1.0, which combines the capabilities of all these tools to provide everyone in the AI research community with a fast path for building a broad range of AI projects. The technology in PyTorch 1.0 is already being used at scale, including performing nearly 6 billion text translations per day for the 48 most commonly used languages on Facebook. In VR, these tools have helped in deploying new research into production to make avatars move more realistically.
The PyTorch 1.0 toolkit will be available in beta within the next few months. With it, developers can take advantage of computer vision advances like DensePose, which can put a full polygonal mesh overlay on people as they move through a scene.
AR/VR
Facebook's executives also talked about advancements in AR and VR.
The company's research scientists have created a prototype system that can generate 3D reconstructions of physical spaces with "surprisingly convincing" results.
Realistic surroundings are important for creating more immersive AR/VR, but so are realistic avatars. Facebook's teams have been working on research to help computers generate photorealistic avatars, seen below.
Connectivity
These advances in AI and AR/VR are relevant only if you have access to a strong internet connection - and there are currently 3.8 billion people around the world who don?t have internet access. To increase connectivity around the world, Facebook has focused on developing next-generation technologies that can help bring the cost of connectivity down to reach the unconnected and increase capacity and performance for everyone else. In Uganda, Facebook partnered with local operators to bring new fiber to the region that, when completed, will provide backhaul connectivity covering more than 3 million people and enable future cross-border connectivity to neighboring countries. Meanwhile, Facebook and City of San Jose employees have begun testing an advanced Wi-Fi network supported by Terragraph. Trials of Terragraph are also planned for Hungary and Malaysia. Facebook is also working with hundreds of partners in the Telecom Infra Project to build and launch a variety of efficient network infrastructure solutions