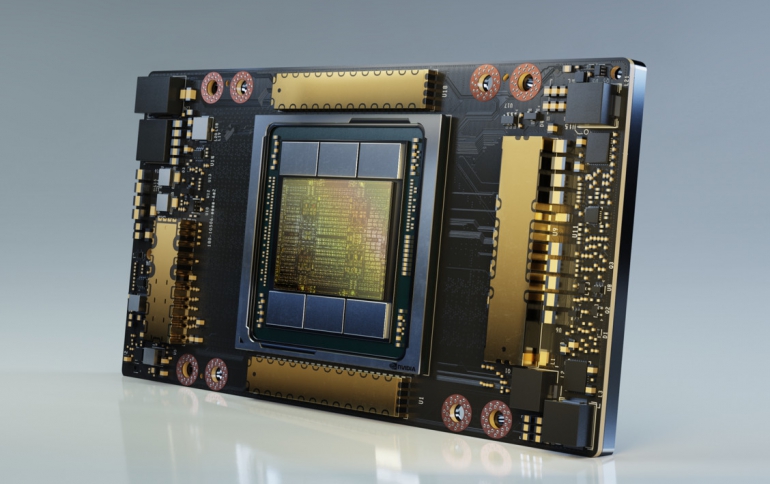
Nvidia Unveils New Ampere Data Center Chips, Ampere Computers, and More
Nvidia announced new graphics chips and computers for artificial intelligence processing in data centers, claiming a leap forward in performance that may help cement its lead in the growing area.
In a keynote delivered in nine simultaneously released episodes recorded from the kitchen of his California home, NVIDIA founder and CEO Jensen Huang discussed NVIDIA’s recent Mellanox acquisition, new products based on the company’s much-awaited NVIDIA Ampere GPU architecture and important new software technologies.
The company’s Ampere chip design is 20 times faster than its predecessors and has the flexibility to be repurposed between the two key areas of artificial intelligence processing: training and inference, Chief Executive Officer Jensen Huang said. The company unveiled the new chip, which Huang called the biggest leap in Nvidia’s history.
And of course, Nvidia CEO confirmed that the chipmaker will use the latest Ampere microarchitecture for all of its next-generation graphics cards.
Nvidia will unify all its products under a single microarchitecture. The prior generation of graphics cards, for example, used two different microarchitectures; Turing for GeForce and Quadro desktop offerings and Volta for the data center Tesla models. That is a thing of the past as the upcoming GeForce, Quadro and Tesla portfolio will all leverage Ampere.
Huang kicked off his keynote on a note of gratitude.
“I want to thank all of the brave men and women who are fighting on the front lines against COVID-19,” Huang said.
NVIDIA, Huang explained, is working with researchers and scientists to use GPUs and AI computing to treat, mitigate, contain and track the pandemic. Among those mentioned:
- Oxford Nanopore Technologies has sequenced the virus genome in just seven hours.
- Plotly is doing real-time infection rate tracing.
- Oak Ridge National Laboratory and the Scripps Research Institute have screened a billion potential drug combinations in a day.
- Structura Biotechnology, the University of Texas at Austin and the National Institutes of Health have reconstructed the 3D structure of the virus’s spike protein.
NVIDIA also announced updates to its NVIDIA Clara healthcare platform aimed at taking on COVID-19.
“Researchers and scientists applying NVIDIA accelerated computing to save lives is the perfect example of our company’s purpose — we build computers to solve problems normal computers cannot,” Huang said.
NVIDIA A100, the first GPU based on the NVIDIA Ampere architecture, provides the greatest generational performance leap of NVIDIA’s eight generations of GPUs, is also built for data analytics, scientific computing and cloud graphics, and is in full production and shipping to customers worldwide, Huang announced.
The new chip can be digitally split up to run several different programs on one physical chip, a first for Nvidia that matches a key capability on many of Intel’s chips.
Owners of data centers will be able to get every bit of computing power possible out of the physical chips they purchase by ensuring the chip never sits idle. The same principle (virtualization) helped power the rise of cloud computing over the past two decades and helped Intel build a massive data center business.
Such virtualization technology came about because software developers realized that powerful and pricey servers often ran far below full computing capacity. By slicing physical machines into smaller virtual ones, developers could cram more software on to them.
Nvidia's new A100 chip can be split into seven “instances.”
Nvidia sells chips for artificial intelligence tasks. The market for those chips breaks into two parts. “Training” requires a powerful chip to, for example, analyze millions of images to train an algorithm to recognize faces. But once the algorithm is trained, “inference” tasks need only a fraction of the computing power to scan a single image and spot a face.
Nvidia is hoping the A100 can replace both, being used as a big single chip for training and split into smaller inference chips.
Eighteen of the world’s leading service providers and systems builders are incorporating them, among them Alibaba Cloud, Amazon Web Services, Baidu Cloud, Cisco, Dell Technologies, Google Cloud, Hewlett Packard Enterprise, Microsoft Azure and Oracle.
Huang detailed five key features of A100, including:
- More than 54 billion transistors, making it the world’s largest 7-nanometer processor.
- Third-generation Tensor Cores with TF32, a new math format that accelerates single-precision AI training out of the box. NVIDIA’s widely used Tensor Cores are now more flexible, faster and easier to use, Huang explained.
- Structural sparsity acceleration, a new efficiency technique harnessing the inherently sparse nature of AI math for higher performance.
- Multi-instance GPU, or MIG, allowing a single A100 to be partitioned into as many as seven independent GPUs, each with its own resources.
- Third-generation NVLink technology, doubling high-speed connectivity between GPUs, allowing A100 servers to act as one giant GPU.
First introduced in the NVIDIA Volta architecture, NVIDIA Tensor Core technology has brought speedups to AI, bringing down training times from weeks to hours and providing massive acceleration to inference. The NVIDIA Ampere architecture builds upon these innovations by bringing new precisions—Tensor Float (TF32) and Floating Point 64 (FP64)—to accelerate and simplify AI adoption and extend the power of Tensor Cores to HPC.
TF32 works just like FP32 while delivering speedups of up to 20X for AI without requiring any code change. Using NVIDIA Automatic Mixed Precision, researchers can gain an additional 2X performance with automatic mixed precision and FP16 adding just a couple of lines of code. And with support for bfloat16, INT8, and INT4, Tensor Cores in NVIDIA A100 Tensor Core GPUs create a versatile accelerator for both AI training and inference. Bringing the power of Tensor Cores to HPC, A100 also enables matrix operations in full, IEEE-certified, FP64 precision.
Every AI and HPC application can benefit from acceleration, but not every application needs the performance of a full A100 GPU. With MIG, each A100 can be partitioned into as many as seven GPU instances, fully isolated and secured at the hardware level with their own high-bandwidth memory, cache, and compute cores. Now, developers can access acceleration for all their applications, big and small, and get guaranteed quality of service. And IT administrators can offer right-sized GPU acceleration for optimal utilization and expand access to every user and application across both bare-metal and virtualized environments.
Scaling applications across multiple GPUs requires extremely fast movement of data. The third generation of NVIDIA NVLink in A100 doubles the GPU-to-GPU direct bandwidth to 600 gigabytes per second (GB/s), almost 10X higher than PCIe Gen4. When paired with the latest generation of NVIDIA NVSwitch, all GPUs in the server can talk to each other at full NVLink speed for incredibly fast data transfers.
Modern AI networks are big and getting bigger, with millions and in some cases billions of parameters. Not all of these parameters are needed for accurate predictions and inference, and some can be converted to zeros to make the models “sparse” without compromising accuracy. Tensor Cores in A100 can provide up to 2X higher performance for sparse models. While the sparsity feature more readily benefits AI inference, it can also be used to improve the performance of model training.
A100 is also bringing massive amounts of compute to data centers. To keep those compute engines fully utilized, it has a leading class 1.6 terabytes per second (TB/sec) of memory bandwidth, a 67 percent increase over the previous generation. In addition, A100 has significantly more on-chip memory, including a 40 megabyte (MB) level 2 cache—7X larger than the previous generation—to maximize compute performance.
The result of all this: 6x higher performance than NVIDIA’s previous generation Volta architecture for training and 7x higher performance for inference.
Ampere chips are already part of servers shipped by Nvidia. The company is pitching them as being capable of replacing much bigger, more expensive and power-hungry racks of machinery that feature a lot of Intel processors.
Third-Generation DGX
Nvidia is selling Ampere-based computers called DGX for $199,000 each. In a typical data center, five of those machines would outperform 600 servers based on Intel chips and the previous generation of Nvidia products, an array that would have cost $11 million, according to Nvidia.
The NVIDIA DGX A100 is the world’s first 5-petaflops server. And each DGX A100 can be divided into as many as 56 applications, all running independently.
Among initial recipients of the system are the U.S. Department of Energy’s Argonne National Laboratory, which will use the cluster’s AI and computing power to better understand and fight COVID-19; the University of Florida; and the German Research Center for Artificial Intelligence.
A100 will also be available for cloud and partner server makers as HGX A100.
A data center powered by five DGX A100 systems for AI training and inference running on just 28 kilowatts of power costing $1 million can do the work of a typical data center with 50 DGX-1 systems for AI training and 600 CPU systems consuming 630 kilowatts and costing over $11 million, Huang explained.
“The more you buy, the more you save,” Huang said, in his common keynote refrain.
DGX A100 systems integrate eight of the new NVIDIA A100 Tensor Core GPUs, providing 320GB of memory for training the largest AI datasets, and the latest high-speed NVIDIA Mellanox HDR 200Gbps interconnects.
Multiple smaller workloads can be accelerated by partitioning the DGX A100 into as many as 56 instances per system, using the A100 multi-instance GPU feature.
DGX A100 Technical Specifications
- Eight NVIDIA A100 Tensor Core GPUs, delivering 5 petaflops of AI power, with 320GB in total GPU memory with 12.4TB per second in bandwidth.
- Six NVIDIA NVSwitch interconnect fabrics with third-generation NVIDIA NVLink technology for 4.8TB per second of bi-directional bandwidth.
- Nine Mellanox ConnectX-6 HDR 200Gb per second network interfaces, offering a total of 3.6Tb per second of bi-directional bandwidth.
- Mellanox In-Network Computing and network acceleration engines such as RDMA, GPUDirect and Scalable Hierarchical Aggregation and Reduction Protocol (SHARP) to enable the highest performance and scalability.
- 15TB Gen4 NVMe internal storage, which is 2x faster than Gen3 NVMe SSDs.
- NVIDIA DGX software stack, which includes optimized software for AI and data science workloads, delivering maximized performance and enabling enterprises to achieve a faster return on their investment in AI infrastructure.
A single rack of five DGX A100 systems replaces a data center of AI training and inference infrastructure, with 1/20th the power consumed, 1/25th the space and 1/10th the cost.
Nvidia chips have grabbed market share in the AI area of training, which is the rapid crunching of huge amounts of data -- such as images or sound files -- to determine patterns that are then embedded into software. Inference, running that resulting software to provide real-time responses for things like voice commands, has been mainly done on servers that use Intel Xeon chips.
AMD, Nvidia’s biggest rival in computer gaming graphics chips, is also touting new capabilities for data centers.
These new tools arrive as the effectiveness of machine learning has driven companies to collect more and more data. “That positive feedback is causing us to experience an exponential growth in the amount of data that is collected,” Huang said.
To help organizations of all kinds keep up, Huang announced support for NVIDIA GPU acceleration on Spark 3.0, describing the big data analytics engine as “one of the most important applications in the world today.”
Built on RAPIDS, Spark 3.0 shatters performance benchmarks for extracting, transforming and loading data, Huang said. It’s already helped Adobe Intelligent Services achieve a 90 percent compute cost reduction.
Key cloud analytics platforms — including Amazon SageMaker, Azure Machine Learning, Databricks, Google Cloud AI and Google Cloud Dataproc — will all accelerate with NVIDIA, Huang announced.
“We’re now prepared for a future where the amount of data will continue to grow exponentially from tens or hundreds of petabytes to exascale and beyond,” Huang said.
Huang also unveiled NVIDIA Merlin, an end-to-end framework for building next-generation recommender systems, which are fast becoming the engine of a more personalized internet. Merlin slashes the time needed to create a recommender system from a 100-terabyte dataset to 20 minutes from four days, Huang said.
The latest generation of deep-learning powered recommender systems provide marketing magic, giving companies the ability to boost click-through rates by better targeting users who will be interested in what they have to offer.
Now the ability to collect this data, process it, use it to train AI models and deploy those models to help you and others find what you want is among the largest competitive advantages possessed by the biggest internet companies.
Recommenders work by collecting information — by noting what you ask for — such as what movies you tell your video streaming app you want to see, ratings and reviews you’ve submitted, purchases you’ve made, and other actions you’ve taken in the past
Perhaps more importantly, they can keep track of choices you’ve made: what you click on and how you navigate. How long you watch a particular movie, for example. Or which ads you click on or which friends you interact with.
All this information is streamed into vast data centers and compiled into complex, multidimensional tables that quickly balloon in size.
They can be hundreds of terabytes large — and they’re growing all the time. In other words, these tables are sparse — most of the information most of these services have on most of us for most of these categories is zero. But, collectively these tables contain a great deal of information on the preferences of a large number of people.
NVIDIA GPUs, of course, have long been used to accelerate training times for neural networks — sparking the modern AI boom — since their parallel processing capabilities let them blast through data-intensive tasks.
NVIDIA’s Merlin recommender application framework promises to make GPU-accelerated recommender systems more accessible still with an end-to-end pipeline for ingesting, training and deploying GPU-accelerated recommender systems.
These systems will be able to take advantage of the new NVIDIA A100 GPU, built on the NVIDIA Ampere architecture, so companies can build recommender systems more quickly and economically than ever.
DGX SuperPOD
Huang also announced the next-generation DGX SuperPOD. Powered by 140 DGX A100 systems and Mellanox networking technology, it offers 700 petaflops of AI performance, Huang said, the equivalent of one of the 20 fastest computers in the world.
NVIDIA built the DGX SuperPOD AI supercomputer for internal research in areas such as conversational AI, genomics and autonomous driving.
The cluster is one of the world’s fastest AI supercomputers — achieving a level of performance that previously required thousands of servers. The enterprise-ready architecture and performance of the DGX A100 enabled NVIDIA to build the system in less than a month, instead of taking months or years of planning and procurement of specialized components previously required to deliver these supercomputing capabilities.
To help customers build their own A100-powered data centers, NVIDIA has released a new DGX SuperPOD reference architecture. It gives customers a blueprint that follows the same design principles and best practices NVIDIA used to build its DGX A100-based AI supercomputing cluster.
NVIDIA is expanding its own data center with four DGX SuperPODs, adding 2.8 exaflops of AI computing power — for a total of 4.6 exaflops of total capacity — to its SATURNV internal supercomputer, making it the world’s fastest AI supercomputer.
NVIDIA EGX A100
Huang also announced the NVIDIA EGX A100, bringing real-time cloud-computing capabilities to the edge. Its NVIDIA Ampere architecture GPU offers third-generation Tensor Cores and new security features. Thanks to its NVIDIA Mellanox ConnectX-6 SmartNIC, it also includes secure, fast networking capabilities.
NVIDIA today announced two products for its EGX Edge AI platform — the EGX A100 for larger commercial off-the-shelf servers and the tiny EGX Jetson Xavier NX for micro-edge servers — delivering high-performance, secure AI processing at the edge.
With the NVIDIA EGX Edge AI platform, hospitals, stores, farms and factories can carry out real-time processing and protection of the massive amounts of data streaming from trillions of edge sensors. The platform makes it possible to securely deploy, manage and update fleets of servers remotely.
The EGX A100 converged accelerator and EGX Jetson Xavier NX micro-edge server are created to serve different size, cost and performance needs. Servers powered by the EGX A100 can manage hundreds of cameras in airports, for example, while the EGX Jetson Xavier NX is built to manage a handful of cameras in convenience stores. Cloud-native support ensures the entire EGX lineup can use the same optimized AI software to easily build and deploy AI applications.
The EGX A100 is the first edge AI product based on the NVIDIA Ampere architecture. As AI moves increasingly to the edge, organizations can include EGX A100 in their servers to carry out real-time processing and protection of the massive amounts of streaming data from edge sensors.
It combines the computing performance of the NVIDIA Ampere architecture with the accelerated networking and critical security capabilities of the NVIDIA Mellanox ConnectX-6 Dx SmartNIC to transform standard and purpose-built edge servers into secure, cloud-native AI supercomputers.
With an NVIDIA Mellanox ConnectX-6 Dx network card onboard, the EGX A100 can receive up to 200 Gbps of data and send it directly to the GPU memory for AI or 5G signal processing. With the introduction of NVIDIA Mellanox’s time-triggered transport technology for telco (5T for 5G), EGX A100 is a cloud-native, software-defined accelerator that can handle the most latency-sensitive use cases for 5G.
Jetson Xavier NX Developer Kit with Cloud-Native Support
NVIDIA also announced availability of the NVIDIA Jetson Xavier NX developer kit with cloud-native support — and the extension of this support to the entire NVIDIA Jetson edge computing lineup for autonomous machines.
The Jetson Xavier NX module is a platform designed to accelerate AI applications, delivering greater than 10x higher performance compared to its widely adopted predecessor, Jetson TX2. Using cloud-native technologies, developers can take advantage of the module’s high AI and compute performance in its credit card-sized form factor.
With support for cloud-native technologies now available across the NVIDIA Jetson lineup, manufacturers of intelligent machines and developers of AI applications can build and deploy software-defined features on embedded and edge devices targeting robotics, smart cities, healthcare, industrial IoT and more.
Cloud-native support helps manufacturers and developers implement frequent improvements, improve accuracy and use the latest features with Jetson-based AI edge devices. And developers can deploy new algorithms throughout an application’s lifecycle, at scale, while minimizing downtime.
Developers can work with the models from NGC and the latest NVIDIA tools and optimize directly on a Jetson Xavier NX developer kit or other Jetson developer kits. They can also use a workstation with cross-compile toolchain or even cloud development workflows using containers coming soon to NGC.
The Jetson Xavier NX developer kit is the development platform for the Jetson Xavier NX module. Smaller than a credit card (70x45mm), the energy-efficient module delivers server-class performance — up to 21 TOPS at 15W, or 14 TOPS at 10W.
As a result, Jetson Xavier NX opens the door for embedded edge-computing devices that demand increased performance to support AI workloads but are constrained by size, weight, power budget or cost.
Powered by the NVIDIA CUDA-X accelerated computing stack and Jetpack SDK, which comes with NVIDIA container runtime, the Jetson Xavier NX developer kit combines a reference module and carrier board with a full Linux software development environment.
The Jetson Xavier NX developer kit and the Jetson Xavier NX module are now available through NVIDIA’s distribution channels for $399.
Fully Optimized, Cloud-Native Software Across the EGX Edge AI Platform
The EGX Edge AI platform’s cloud-native architecture allows it to run containerized software to support a range of GPU-accelerated workloads.
NVIDIA application frameworks include Clara for healthcare, Aerial for telcos, Jarvis for conversational AI, Isaac for robotics, and Metropolis for smart cities, retail, transportation and more. They can be used together or individually and open new possibilities for a variety of edge use cases.
With support for cloud-native technologies now available across the entire NVIDIA EGX lineup, manufacturers of intelligent machines and developers of AI applications can build and deploy high-quality, software-defined features on embedded and edge devices targeting robotics, smart cities, healthcare, industrial IoT and more.
Global Support for EGX Ecosystem
Existing edge servers enabled with NVIDIA EGX software are available from global enterprise computing providers Atos, Dell Technologies, Fujitsu, GIGABYTE, Hewlett Packard Enterprise, , Inspur, Lenovo, Quanta/QCT and Supermicro. They are also available from major server and IoT system makers such as ADLINK and Advantech.
These servers along with optimized application frameworks can be used by software vendors such as Whiteboard Coordinator, Deep Vision AI, IronYun, Malong and SAFR by RealNetworks to build and deploy healthcare, retail, manufacturing and smart cities solutions.
The EGX A100 will be available at the end of the year. Ready-to-deploy micro-edge servers based on the EGX Jetson Xavier NX are available now for companies looking to create high-volume production edge systems.
NVIDIA Jarvis
Huang also detailed NVIDIA Jarvis, a new end-to-end platform for creating real-time, multimodal conversational AI that can draw upon the capabilities unleashed by NVIDIA’s AI platform.
Applications built with Jarvis can take advantage of innovations in the new NVIDIA A100 Tensor Core GPU for AI computing and the latest optimizations in NVIDIA TensorRT for inference. For the first time, it’s now possible to run an entire multimodal application, using the most powerful vision and speech models, faster than the 300-millisecond threshold for real-time interactions.
Jarvis provides a complete, GPU-accelerated software stack and tools making it easy for developers to create, deploy and run end-to-end, real-time conversational AI applications that can understand terminology unique to each company and its customers.
To offer an interactive, personalized experience, companies need to train their language-based applications on data that is specific to their own product offerings and customer requirements. However, building a service from scratch requires deep AI expertise, large amounts of data and compute resources to train the models, and software to regularly update models with new data.
Jarvis addresses these challenges by offering an end-to-end deep learning pipeline for conversational AI. It includes deep learning models, such as NVIDIA’s Megatron BERT for natural language understanding. Enterprises can further fine-tune these models on their data using NVIDIA NeMo, optimize for inference using TensorRT, and deploy in the cloud and at the edge using Helm charts available on NGC, NVIDIA’s catalog of GPU-optimized software.
Huang highlighted its capabilities with a demo that showed him interacting with a friendly AI, Misty, that understood and responded to a sophisticated series of questions about the weather in real time.
An early access program for NVIDIA Jarvis is available to a limited number of applicants.
The NVIDIA Mellanox ConnectX-6 Lx SmartNIC
The NVIDIA Mellanox ConnectX-6 Lx SmartNIC is a highly secure and efficient 25/50 gigabit per second (Gb/s) Ethernet smart network interface controller (SmartNIC). ConnectX-6 Lx, the 11th generation product in the ConnectX family, is designed to meet the needs of modern data centers, where 25Gb/s connections are becoming standard for handling demanding workflows, such as enterprise applications, AI and real-time analytics.
Accelerated security features such as IPsec in-line cryptography and Hardware Root of Trust, and a 10x performance improvement for Connection Tracking, enable Zero Trust security throughout the data center. RDMA over converged Ethernet (RoCE), advanced virtualization and containerization, and NVMe over Fabrics storage offloads provide highly scalable and performant networking.
The Mellanox ConnectX-6 Lx provides:
- Two ports of 25Gb/s, or a single port of 50Gb/s, Ethernet connectivity with PCIe Gen 3.0/4.0 x8 host connectivity
- Security features including Hardware Root of Trust, Connection Tracking for stateful L4 firewalls, and in-line IPSec cryptography acceleration
- GPUDirect RDMA acceleration for NVMe over Fabrics (NVMe-oF) storage, scale-out accelerated computing and high-speed video transfer applications
- Zero Touch RoCE (ZTR) for scalable, easy-to-deploy, best-in-class RoCE without switch configuration
- Accelerated switching and packet processing (ASAP2), with built-in SR-IOV and VirtIO hardware offloads for virtualization and containerization, to accelerate software-defined networking and connection tracking for next-generation firewall services
ConnectX-6 Lx is sampling now, with general availability expected in Q3 2020. Like all products in the ConnectX family, it is compatible with the Mellanox SmartNIC software stack.
Autonomous Vehicles
Autonomous vehicles are one of the greatest computing challenges of our time, Huang said, an area where NVIDIA continues to push forward with NVIDIA DRIVE.
NVIDIA DRIVE will use the new Orin SoC with an embedded NVIDIA Ampere GPU to achieve the energy efficiency and performance to offer a 5-watt ADAS system for the front windshield as well as scale up to a 2,000 TOPS, level-5 robotaxi system.
Now automakers have a single computing architecture and single software stack to build AI into every one of their vehicles.
“It’s now possible for a carmaker to develop an entire fleet of cars with one architecture, leveraging the software development across their whole fleet,” Huang said.
The NVIDIA DRIVE ecosystem now encompasses cars, trucks, tier one automotive suppliers, next-generation mobility services, startups, mapping services, and simulation.
This newly expanded range starts at an NCAP 5-star ADAS system and runs all the way to a DRIVE AGX Pegasus robotaxi platform. The latter features two Orin SoCs and two NVIDIA Ampere GPUs to achieve an unprecedented 2,000 trillion operations per second, or TOPS — more than 6x the performance of the previous platform.
The current generation of DRIVE AGX delivers capabilities that scale from level 2+ automated driving to level 5 fully autonomous driving with different combinations of Xavier SoCs and Turing-based GPUs. DRIVE AGX Xavier delivers 30 TOPS of performance and the NVIDIA DRIVE AGX Pegasus platform processes up to 320 TOPS to run multiple redundant and diverse deep neural networks for real-time perception, planning and control.
The new DRIVE AGX family now begins with a single Orin SoC variant that sips just five watts of energy and delivers 10 TOPS of performance.
With a single platform, developers can leverage one architecture to more easily develop autonomous driving technology across all their market segments. And since the DRIVE platform is software-defined and based on the large CUDA developer community, it can easily and constantly benefit from over-the-air updates.
With two Orin SoCs and two NVIDIA Ampere-based GPUs delivering 2,000 TOPS, the platform is capable of handling higher resolution sensor inputs and more advanced autonomous driving DNNs required for full self-driving robotaxi operation.
The architecture offers the largest leap in performance within the eight generations of NVIDIA GPUs — boosting performance by up to 6x.
The Orin family of SoCs will begin sampling next year and be available for automakers starting production in late 2022, laying the foundation for the next-generation of the programmable, software-defined NVIDIA DRIVE AGX lineup.
Nvidia declined to comment on pricing or potential automaker customers. However, he said the Nvidia chips will be part of a larger system that includes cameras and will likely be built by traditional automotive suppliers such as Continental AG, ZF Friedrichshafen AG or Robert Bosch.
And Huang announced NVIDIA is adding NVIDIA DRIVE RC for managing entire fleets of autonomous vehicles to its suite of NVIDIA DRIVE technologies.
Robotics
NVIDIA also continues to push forward with its NVIDIA Isaac software-defined robotics platform, announcing that BMW has selected NVIDIA Isaac robotics to power its next-generation factories.
BMW’s 30 factories around the globe build one vehicle every 56 seconds: that’s 40 different models, each with hundreds of different options, made from 30 million parts flowing in from nearly 2,000 suppliers around the world, Huang explained.
BMW joins a sprawling NVIDIA robotics global ecosystem that spans delivery services, retail, autonomous mobile robots, agriculture, services, logistics, manufacturing and healthcare.
In the future, factories will, effectively, be enormous robots. “All of the moving parts inside will be driven by artificial intelligence,” Huang said. “Every single mass-produced product in the future will be customized.”