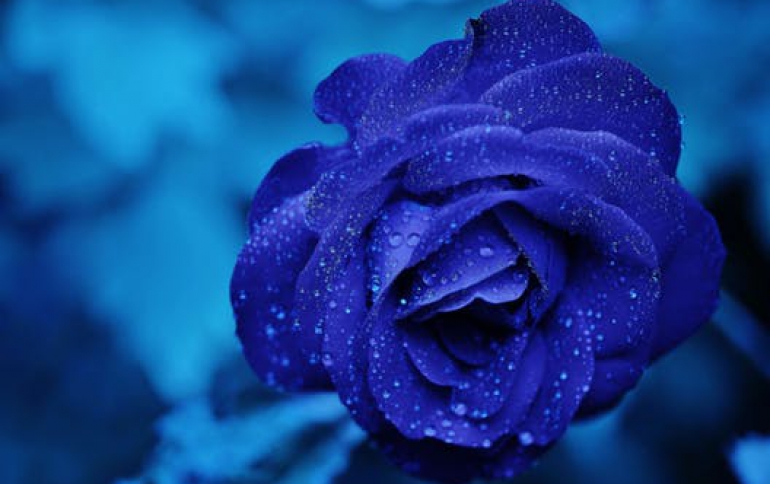
New Deep Fujitsu Learning Technology Analyzes Time-Series Data With High Precision
Fujitsu Laboratories has developed deep learning technology that can accurately analyze time-series data. Deep learning technology (a type of machine learning using a multi-layered neural-network model) is attracting attention as a breakthrough in the advance of artificial intelligence. The technology has achieved extremely high recognition accuracy with images and speech, but the types of data to which it can be applied is still limited.
In particular, for complex time-series data that is subject to severe oscillations and captured by sensors embedded in IoT devices, it has so far been difficult to achieve highly accurate classifications using deep learning or any other machine learning techniques.
Fujitsu Laboratories has developed deep learning technology that uses advanced chaos theory and topology to automatically and accurately classify volatile time-series data. This can accurately handle even complex time-series data with severe oscillations.
The technology uses the following procedures to learn and classify:
- Numerical data captured by sensors are represented superficially as the product of a complex combination of dynamic movements. While it is difficult to directly investigate the mechanisms of these dynamic movements, plotting changes over time on a graph is known to reveal characteristic tracks for each mechanism of movement. Applying this graphic approach makes it possible to distinguish between the time-series data via diagrams.
- Because it is difficult to directly apply machine learning to the diagrams created in step 1, Fujitsu Laboratories applied topological data analysis to express features of the diagrams as numbers. The topological data analysis is a data-analysis technique that treats data as a set of points arrayed within a certain space, from which geometric information can be extracted. With this method, rather than the features one might normally associate with a graphical image, the number of holes contained in the diagram and the major shape features are analyzed and then converted into a propriety vector representation.
- Fujitsu Laboratories newly designed a convolutional neural network that trains on the propriety vector representations from step 2, enabling the classification of volatile time-series data.
Using the time-series data from gyroscopic sensors built into wearable devices, this technology was found to achieve an accuracy of approximately 85%, an improvement of about 25% over existing techniques, on the UC Irvine Machine Learning Repository benchmark test for classifying human activities. In tests to infer mental states using brainwave time-series data, this technique achieved an accuracy of about 77%, a roughly 20% improvement over existing techniques.
This technology extends the types of data to which deep learning can be applied to time-series data. Moreover, because it can very accurately classify time-series data with severe oscillations, which people have difficulty discerning, it enables new types of analyses. For example, using IoT devices, it could be used to accurately detect equipment anomalies or forecast breakdowns in factories, or could be used to analyze vital-signs data to assist with medical diagnoses and treatment. In ways such as these, it is expected that this technology will bring advancements to a variety of fields through artificial intelligence.
Fujitsu Laboratories will work on further improving the accuracy of its time-series data classification technology with the aim of a practical implementation of it in fiscal 2016. The company is also working to broaden the application of deep learning to types of data other than images, sounds, and time series, and to perform more sophisticated data analysis.
Details of this technology will be presented at the Fujitsu North America Technology Forum (NAFT 2016), which will be held on Tuesday, February 16, in Santa Clara, California.